A Cancer Genome Interpreter to identify driver and actionable alterations in tumors
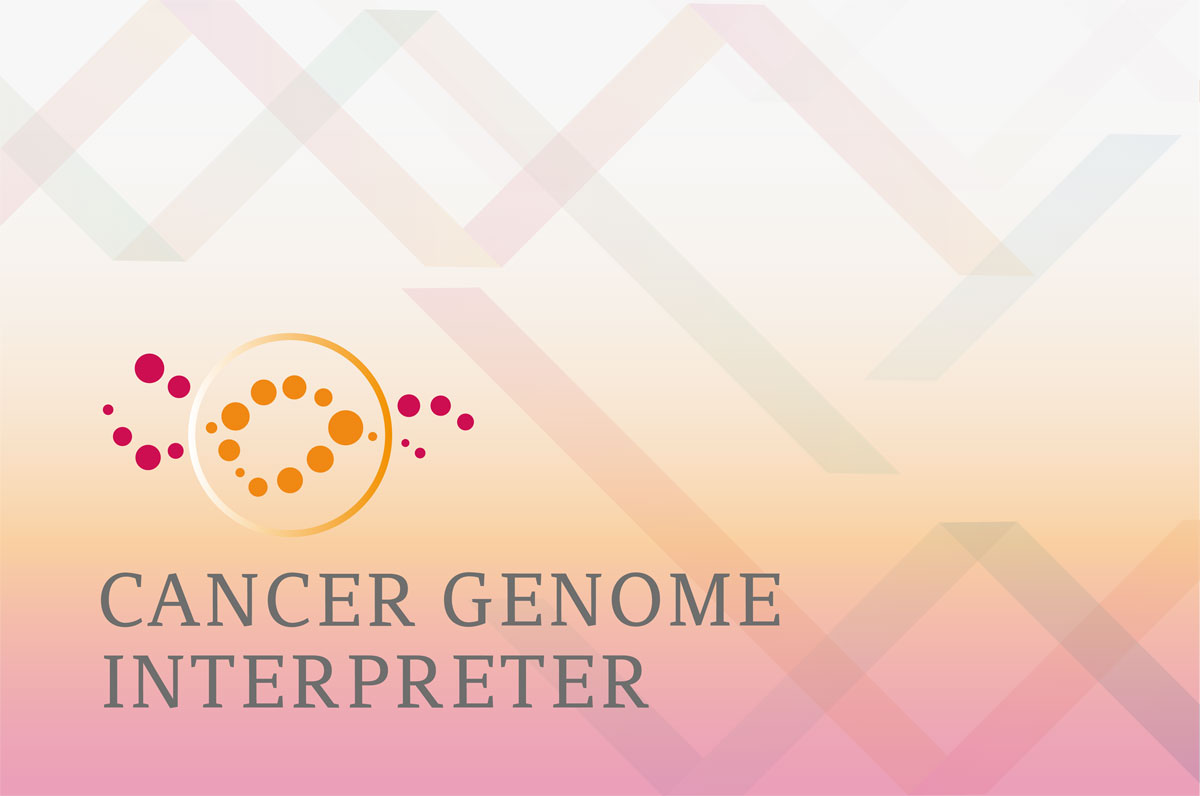
The use of genomic information is becoming a key piece of the oncology toolkit to make informed decisions aimed to improve the management of the disease and increase the cost-effectiveness of available therapies. Although in recent years the relevance of many oncogenic alterations in malignant transformation has been identified and validated across cancer types, the relevance for cancer growth of most alterations in a patient’s tumor are still of uncertain significance and their usefulness to inform the most appropriate treatment is unclear. Furthermore, mounting experimental and clinical data on tumor alterations driving the disease and influencing the response to anti-cancer therapies is currently gathered across scattered and fragmented resources, annotated with dissimilar approaches and with no easy framework to match the knowledge they store with the alterations observed in a patient’s tumor. These problems severely limit the value that the genomic information of a tumor individual provides beyond the well-known biomarkers of drug response.
To address these difficulties we have developed the Cancer Genome Interpreter (CGI, http://www.cancergenomeinterpreter.org), which supports the biological and clinical interpretation of the alterations (mutations, copy number alterations and/or translocations) found in a patient’s tumor according to different levels of relevance. The outcomes of the platform are of broad utility in both translational and pre-clinical settings. Briefly, and upon reception of the list of alterations detected in a tumor, the CGI first identifies already validated oncogenic events. Second, it predicts the effect of the remaining alterations of uncertain significance by using an ensemble of bioinformatics methods. Of note, the evaluation of single nucleotide changes and small indels is carried out by OncodriveMUT, a novel method developed within the CGI to estimate the oncogenic potential of mutations. This method combines mutation-centric measurements with features characterizing the genes (or regions within genes) where the mutations occur, derived from the analysis of cohorts of sequenced tumors –as the identification of gene regions exhibiting somatic mutation clusters– and samples from healthy donors –as the identification of protein domains depleted by functional germline variants. Third, the CGI reports the influence of these variants on the clinical response to drugs (in terms of sensitivity, resistance and toxicity) according to the current state of knowledge manually curated by a board of oncologists (ranging from pre-clinical evidences to clinical guidelines). Finally, the CGI lists the available interactions of existing chemical compounds with all genes bearing driver alterations in the tumor sample with the aim to explore novel actionable events.
The results of CGI analyses are provided via interactive reports that (a) are structured to fulfil the needs of each use case; and (b) comprise the ancillary data in which the system bases its classifications to support researchers in the review of the platform outputs. Importantly, both genomic alteration analysis and the in silico prescription reports are organized according to different levels of clinical relevance: in one side, the identification of driver events can be divided between those alterations already validated as being oncogenic in one or more tumor types versus those whose effect is unknown and thus is predicted by using the ensemble of bioinformatics methods included in the CGI pipeline. On the other side, the in silico prescription is divided between the detection of biomarkers of drug response that have been observed in tumor patients or in pre-clinical cancer assays (cancer bioMarkersDB) versus the compilation of gene target-chemical compounds interaction data retrieved from bioactivity assays (cancer bioActivityDB). The former is further divided according to the level of evidence supporting the use of each biomarker (ranged from pre-clinical data to standard-of-care guidelines). It also indicates whether the described biomarker matches completely the observed alteration and the tumor type of the sample can be used as a novel repurposing opportunity. These comprehensive and versatile outputs should position the CGI as a tool of broad utility for both pre-clinical and translational oncology settings. We envisage that the CGI will support decisions in different scenarios of precision medicine efforts, such as the selection of the most appropriate clinical trial for patients whose tumor exhibit mutations of unknown significance or the design of experimental assays aimed to evaluate the use of novel genomic guided-therapeutic strategies.
A critical issue in the CGI is the maintenance of high quality data and the improvement of the predictive methods. The aggregation, curation and interpretation of the in-house databases used by the CGI follows the standard operating procedures developed under the umbrella of the EU’s Horizon 2020 MedBioinformatics project that ensures its mid-term maintenance, and the feedback of the community is also allowed through the CGI web interface. However, the availability of data of such nature is both key for the advance of cancer precision medicine and difficult to address for an individual institution. For this reason, the Variant Interpretation for Cancer Consortium working group under the Global Alliance for Genomics & Health framework has been recently launched with the aim of unifying the curation efforts currently ongoing in several institutes, including ours. Thus, as data on novel biomarkers for alternative therapeutic approaches, such as combination of targeted drugs or immunotherapies become available, these will be incorporated to the CGI. Of note, known effects of interacting genomic events affecting the response to certain drugs are already included. Similarly, the performance of the bioinformatics predictions will improve as new data is generated to feed the in silico methods and novel features are incorporated to the arsenal of oncogenic proxies. We envisage that although individual databases will continue to exist to fulfil specific needs, the long-term outcome of the CGI platform will largely rely on the existence of standards to gather the cancer variant-clinical outcome associations and the success in engaging the community for sharing such knowledge.